Overview
Data-driven insights are the lifeblood of modern healthcare delivery. They fuel complex machine learning platforms, behavioral analytics for patient experience, and countless other innovations disrupting the industry. Healthcare data engineering plays a pivotal role in unlocking these insights, but it comes with unique challenges.
Healthcare Data Engineering Challenges:
- Big data complexities: From high-velocity IoT data to massive genomics and radiology datasets, healthcare presents a unique big data challenge.
- Compliance and regulations: Managing evidence-based clinical guidelines, administrative rules, and regulatory submission standards adds another layer of complexity.
- Data security: Protecting sensitive patient information is paramount, requiring robust security measures.
Cybage: Your Partner in Healthcare Data Engineering Success
With extensive experience across industries, Cybage offers comprehensive data engineering consulting services and solutions specifically tailored to the healthcare landscape. We understand the unique challenges you face and provide a multifaceted approach that includes:
- Data engineering platform implementation: We help you leverage traditional data warehouses, modern data lakes, and cutting-edge DevOps practices to build a robust data infrastructure.
- ETL lifecycle management: Our expertise ensures efficient data extraction, transformation, and loading processes, ensuring data quality and accessibility.
- 24/7 support model: We provide ongoing support to ensure your data engineering solutions function flawlessly and evolve with your needs.
By partnering with Cybage, you gain access to a team of experienced data engineers who can help you:
- Turn raw data into actionable insights.
- Optimize clinical decision-making.
- Enhance patient experience.
- Navigate complex compliance requirements.
- Ensure data security and privacy.
Unlock the transformative power of healthcare data engineering with Cybage!
Our Expertise
ETL (Extract, Transform, Load) extracting data from various sources such as electronic health records (EHRs), medical imaging systems, pharmacy systems, and lab systems; transforming the data into a standardized format; and loading it into a data warehouse or another storage system for analysis and reporting.
Data modeling in healthcare involves designing the structure of databases and data warehouses to represent and store healthcare-related information accurately. It includes patient records, medical histories, treatment plans, and more.
Essential for data-driven decision-making and improving patient care, optimization with strategies like incremental loading, parallel processing, data compression, indexing, data partitioning, and monitoring and tuning.
Semantic transformation standardizes and harmonizes data from different sources to be easily understood and shared. For example, it is mapping different coding systems (e.g., ICD-10, SNOMED CT) to a common terminology to ensure consistency in data representation. Syntactic transformations convert data between various file formats or communication protocols. For example, data can be converted from HL7 v2 to HL7 v3 format for interoperability between healthcare systems.
Transforming complex healthcare real-time data into easily understandable visual representations, such as charts, graphs, and dashboards. It includes patient health monitoring, healthcare performance indicators, and public health surveillance.
Resource Centre
Let's Get Moving!
Your purpose, our passion. Connect with us and let's make things happen.
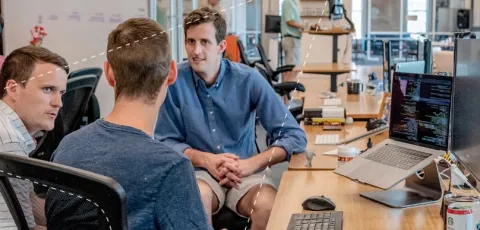